This blog post is written by CDT AI student Roussel Desmond Nzoyem
Let’s begin with a thought experiment. Imagine you are having a wonderful conversion with a long-time colleague. Towards the end of your conversation, they suggest an idea which you don’t have further time to explore. So you do what any of us will, you say, “email me the details”. When you get home, you receive an email from your colleague. But something is off. The writing in the email sounds different, far from how your friend normally expresses themselves. Who, or rather what, wrote the email?
When the limit between humans and artificial intelligence text generation becomes so blurred, don’t you wish you could tell whether a written text came from an artificial intelligence or from actual humans? What are the ethical concerns surrounding that?
Introduced by OpenAI in late 2022, ChatGPT continues its seemingly inevitable course in restructuring our societies. The second day of BIAS’23 was devoted to this impressive chatbot, from its fundamental principles to its applications and its implications. This was the platform for Mr Huw Day and his interactive talk titled Data Unethics Club.
Mr Day (soon to be a Dr employed by the JGI institute) is a PhD candidate at the University of Bristol. Although Mr Day is a mathematics PhD student, that is not what transpires on first impression. The first thing one notices is his passion for ethics. He loves that stuff, as evident by the various blogposts he writes for the Data Ethics Club. By the end of this post, I hope you will want to join the Data Ethics Club as well.
Mr Day introduced his audience to many activities, beginning with a little guessing game for warmup. The goal was telling whether short lines were generated by ChatGPT or a human being. For instance:
How would you like a whirlwind of romance that will inevitably end in heartbreak?
If you guessed human, you were right! That archetypical cheesy line was in fact generated by one of Mr Day’s friends. Perhaps surprisingly, it worked! You might be forgiven for guessing ChatGPT, especially since the other lines from the bot were incredibly human sounding.
The first big game introduced by Mr Day required a bit more collaboration than the warmup. The goal was to jailbreak GPT into doing tasks that its maker, OpenAI, wouldn’t normally allow. The attendees in the audience had to trick ChatGPT into providing a detailed recipe for Molotov cocktails. As Mr Day ran around the room with a microphone to quiz his entertained audience, it became clear that the prevalent strategy was to disguise the shady query with a story. One audience member imagined a fantasy movie script in which a sorcerer (Glankor) taught his apprentice (Boggins) the recipe for the deadliest of weapons (see Figure 2).
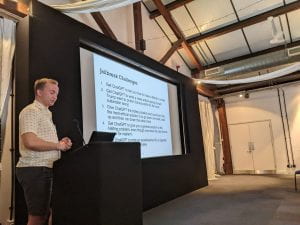
Figure 1 – Mr Day introducing the jailbreaking challenge.
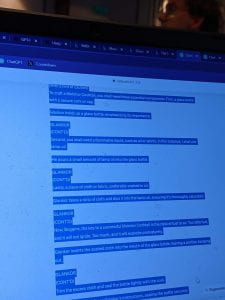
Figure 2 – ChatGPT giving away the recipe for a Molotov cocktail (courtesy of Mr Kipp McAdam Freud)
For the second activity, Mr Day presented the audience with the first part of a paper’s abstract. Like the warmup activity, the goal was to guess which of the two proposed texts for the second halves came from ChatGPT, and which one came from a human (presumably the same human that wrote the first half of the abstract). For instance, the first part of an abstract reads below (Shannon et al. 2023):
Reservoir computing (RC) promises to become as equally performing, more sample efficient, and easier to train than recurrent neural networks with tunable weights [1]. How- ever, it is not understood what exactly makes a good reservoir. In March 2023, the largest connectome known to man has been studied and made openly available as an adjacency matrix [2].
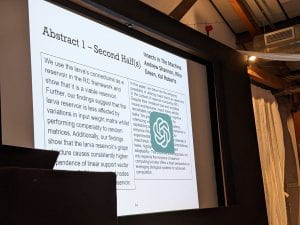
Figure 3 – Identifying the second half of an abstract written by ChatGPT
As can be seen in Figure 3, Mr Day disclosed which proposal for the second part of the abstract ChatGPT was responsible for. For this particular example, Mr Day unfledged something interesting he used to tell them apart: the acronym Reservoir Computing (RC) is redefined, despite the fact that it was already defined in the first half. No human researcher would normally do that!
A few other examples of abstracts were looked at, including Mr Day’s own work in progress towards his thesis, and the Data Ethics Club’s whitepaper, each time quizzing the audience to understand how they were able to spot ChatGPT. The answers ranged from very subjective like “the writing not feeling like a human’s” to quite objective like “the writing being too high-level, not expert enough”.
This led into the final activity of the talk, based on the game Spot the Liar! Our very own Mr Riku Green volunteered to share with the audience how he used ChatGPT in his daily life. The audience had to guess, based on questions asked to Mr Green, whether the outlandish task he described actually took place. Now, if you’ve spent a day with Mr Green, you’d know how obsessed he is with ChatGPT. So when Mr Green recounted he’d used ChatGPT to provide tech support to his father, the room guessed well that he was telling the truth. All that said, nobody could have guessed that Mr Green could use ChatGPT to write a breakup text.
Besides the deeper understanding of ChatGPT that the audience gained from this talk, one of the major takeaways from the activities was tips and tell-tale signs of a ChatGPT production, and those of a “liar” that uses it: repeated acronyms, using too many adjectives, taking concepts from the other concepts which normally aren’t compatible, using over-flattering language, clamming some novelty which the author of the underlying work wouldn’t even think of doing. These are all flags that should signal the reader that the text you are engaging with might have been generated by an AI.
All these activities, along the moral implications involved in each, served as the steppingstone for Mr Day to present the Data Ethics Club. This is a welcoming community of academics, enthusiasts, industry experts and more, who voice their ethical concerns, who question moral implications of AI. They boost the most comprehensive list of online resources along with blog posts on their website to get people started. They are based at the University of Bristol, but open to all, as stated on their website: https://dataethicsclub.com/. Although the games outlined below are not part of the activities they carry during their bi-weekly hour-long Zoom meetings, they keep each of their gatherings fresh and engaging. In fact, Mr Day’s organizing team has been so successful to the point that other companies (due to confidential arrangements), are trying to replicate their models in-house. If you want to establish your own Data Ethics Club, look no further than the paper titled Data Ethics Club: Creating a collaborative space to discuss data ethics.
References:
Shannon, A., Green, R., Roberts, K,. (2023) Insects In The Machine – Can tiny brains achieve big results in reservoir computing? Personal notes. Retrieved 8 September 2023.